编者按:这篇博文是与 Neon 团队(特别是 Raouf Chebri)合作撰写的。向量数据库领域正蓬勃发展,我们很高兴重点介绍新的实现和选项。我们也非常高兴看到这里进行的详细分析,为这个新兴领域带来了一些可靠的统计数据和见解。
我们非常高兴地宣布 Neon 与 LangChain 合作发布 pg_embedding 扩展和 LangChain 中的 PGEmbedding 集成,用于 Postgres 中的向量相似性搜索。
但是等等。LangChain 中不是已经有两个使用 Postgres 和 PGVector 的向量存储了吗?为什么 Neon 团队又增加了一个?
简短的回答是:Neon 团队构建并添加它是为了更快的执行时间和可扩展的 LLM 应用。
PGVector 很棒,它默认执行精确相似性搜索,从而实现 100% 的准确率(召回率)。然而,在大规模情况下,精确搜索成本很高。Neon 发现你可以将 PGVector 与 IVFFlat 索引一起使用来提高查询执行时间,但这通常会以牺牲准确率为代价,从而增加幻觉的可能性。
Neon 团队进行了基准测试,比较了 pgvector 和 PGEmbedding 的性能,他们发现 PGEmbedding 在 99% 的准确率下性能快 20 倍。
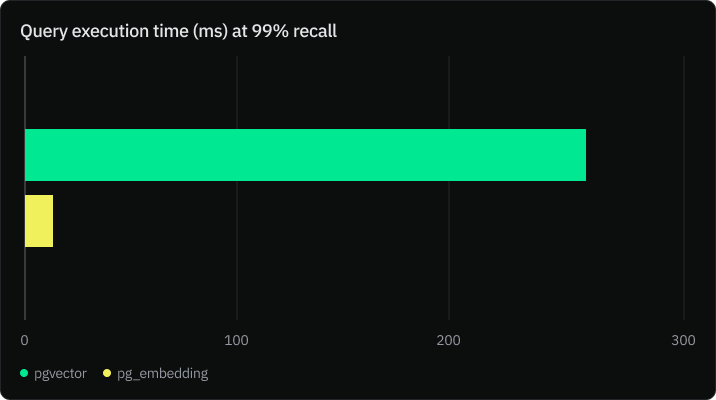
阅读完整文章以了解有关基准的更多信息,请点击此处。
为什么 PGEmbedding 更快?
PGEmbedding 集成使用分层可导航小世界 (HNSW) 索引基于图的方法来索引高维数据。它构建了一个图的层次结构,其中每一层都是前一层的一个子集,这导致时间复杂度为 O(log(rows))。然而,使用 IVFFlat 最佳参数的搜索通常具有 O(sqrt(rows)) 的时间复杂度。
如何开始使用 PGEmbedding
- 第一步是登录你的 Neon 帐户并创建一个项目
npx neonctl auth
如果你还没有 Neon 帐户,上面的命令将引导你进行注册。
登录后,使用以下命令创建一个项目
npx neonctl projects create
预期输出
┌─────────────────┬─────────────────┬───────────────┬──────────────────────┐
│ Id │ Name │ Region Id │ Created At │
├─────────────────┼─────────────────┼───────────────┼──────────────────────┤
│ dawn-sun-749604 │ dawn-sun-749604 │ aws-us-east-2 │ 2023-07-11T20:55:32Z │
└─────────────────┴─────────────────┴───────────────┴──────────────────────┘
┌───────────────────────────────────────────────────────────────────────────────────────┐
│ Connection Uri │
├───────────────────────────────────────────────────────────────────────────────────────┤
│ postgres://<user>:<password>@ep-lingering-moon-792025.us-east-2.aws.neon.tech/neondb │
└───────────────────────────────────────────────────────────────────────────────────────┘
2. 按照文档中的说明安装 LangChain(如果你尚未安装)。
3. 以下代码初始化 PGEmbedding 向量存储,并执行相似性分析
import os
from typing import List, Tuple
from langchain.embeddings.openai import OpenAIEmbeddings
from langchain.text_splitter import CharacterTextSplitter
from langchain.vectorstores import PGEmbedding
from langchain.document_loaders import TextLoader
from langchain.docstore.document import Document
loader = TextLoader('state_of_the_union.txt')
raw_docs = loader.load()
text_splitter = CharacterTextSplitter(chunk_size=1000, chunk_overlap=0)
docs = text_splitter.split_documents(raw_docs)
embeddings = OpenAIEmbeddings()
CONNECTION_STRING = os.environ["DATABASE_URL"]
# Initialize the vectorstore, create tables and store embeddings and
# metadata.
db = PGEmbedding.from_documents(
embedding=embeddings,
documents=docs,
collection_name="state_of_the_union",
connection_string=CONNECTION_STRING,
)
# Create the index using HNSW. This step is optional. By default the
# vectorstore uses exact search.
db.create_hnsw_index(max_elements=10000, dims=1536, m=8, ef_construction =16, ef_search=16)
# Execute the similarity search and return documents
query = "What did the president say about Ketanji Brown Jackson"
docs_with_score = db.similarity_search_with_score(query)
print('query done')
print("Results:")
for doc, score in docs_with_score:
print("-" * 80)
print("Score: ", score)
print(doc.page_content)
print("-" * 80)
PGEmbedding vs PGVector:你应该选择哪个向量存储?
Neon 团队使用五个标准比较了这两个索引
- 搜索速度
- 准确率
- 内存使用
- 索引构建速度
- 距离度量
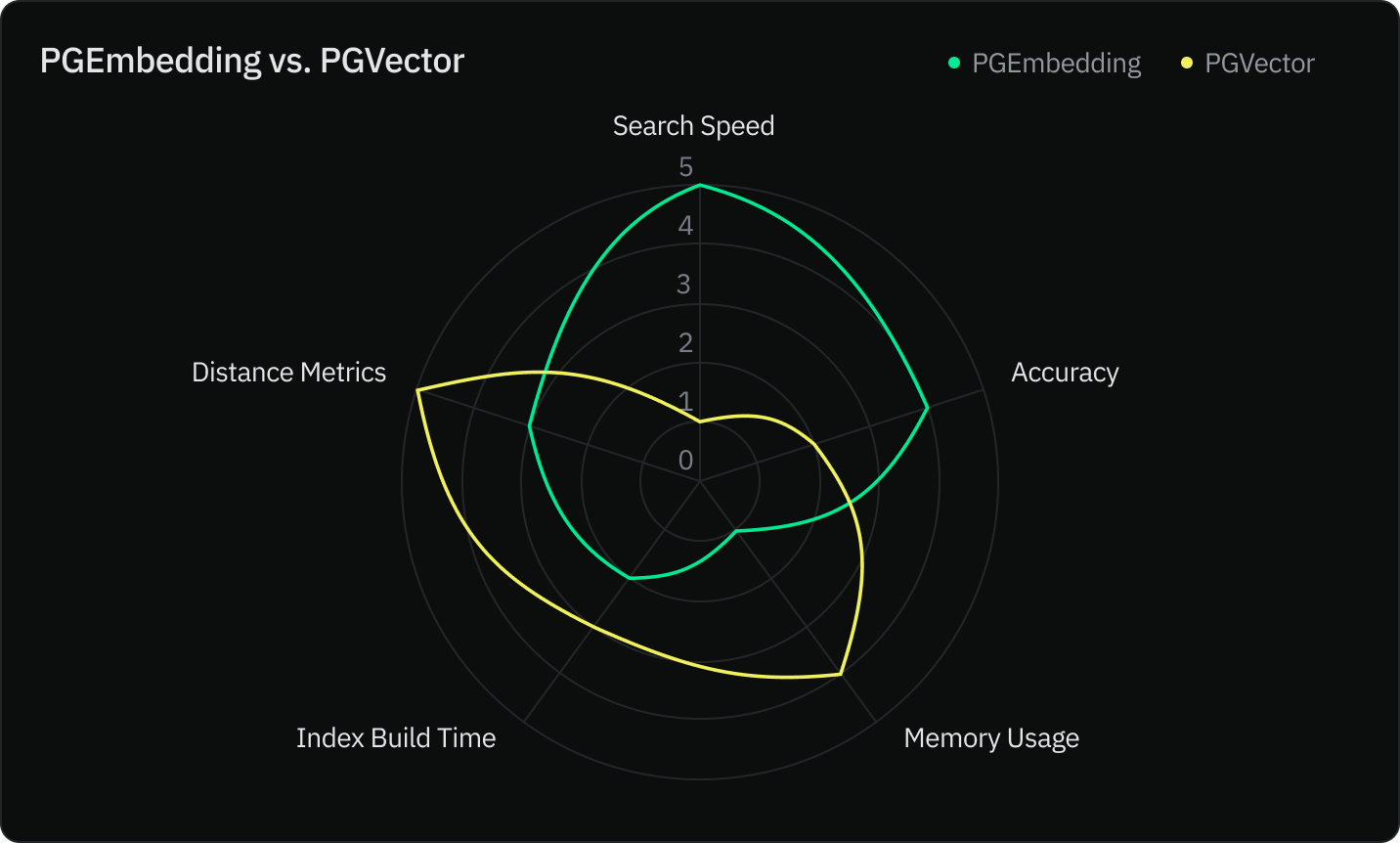
结论
随着 PGEmbedding 集成的推出,你现在拥有了一个强大的新工具来处理你的 LLM 应用程序。 PGVector 对于具有严格内存约束但以牺牲召回率为代价的应用程序仍然是一个可行的选择。
最终,PGEmbedding 和其他向量存储之间的选择应根据你的应用程序的具体需求来决定。我们鼓励你尝试这两种方法,找到最适合你需求的方法。
我们很高兴看到你将使用 PGEmbedding 构建什么,并期待你的反馈!
编码愉快!